Welcome to
Open Invited Tracks
for TC6.3 of IFAC WC2023
in Yokohama、Japan
We are organizing TC6.3 Open Invited Tracks for IFAC World Congress 2023 in Yokohama, Japan, July 9-14, 2023, where TC6.3 stands for IFAC Technical Committee on Power & Energy.
Specifically, the title and proposers of the Open Invited Tracks are given as follows::
#71. Advanced Evolutionary Computation for Operation and Planning in Distribution Networks
(Proposers: Hiroyuki Mori, Meiji Univ., Tokyo, Japan & Hirotaka Takano, Gifu Univ., Gifu, Japan)
#72. Applications of Advanced Deep Neural Networks to Forecasting Problems in Smart Grid
(Proposers: Hiroyuki Mori & Shoichi Urano, Meiji Univ., Tokyo, Japan)
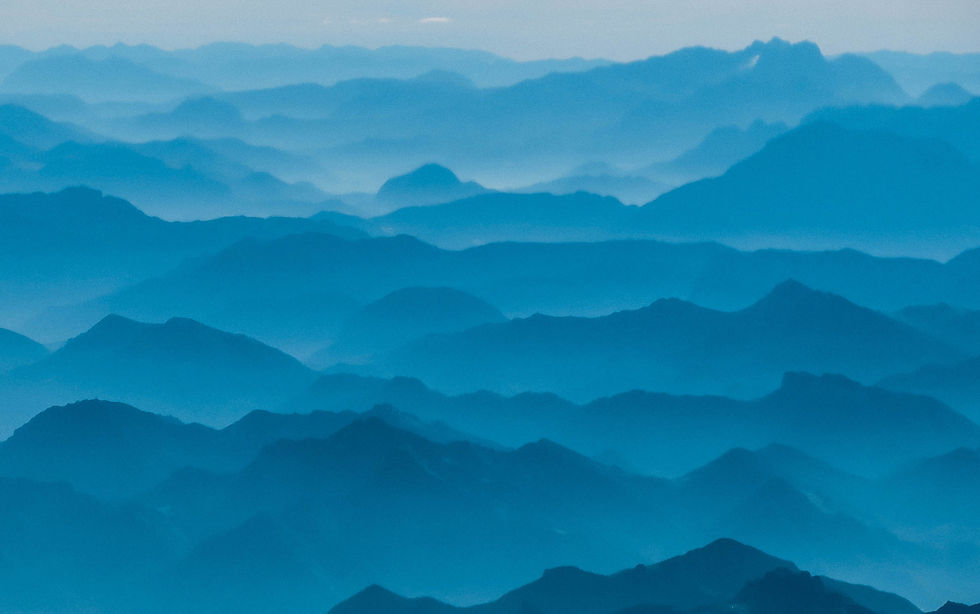



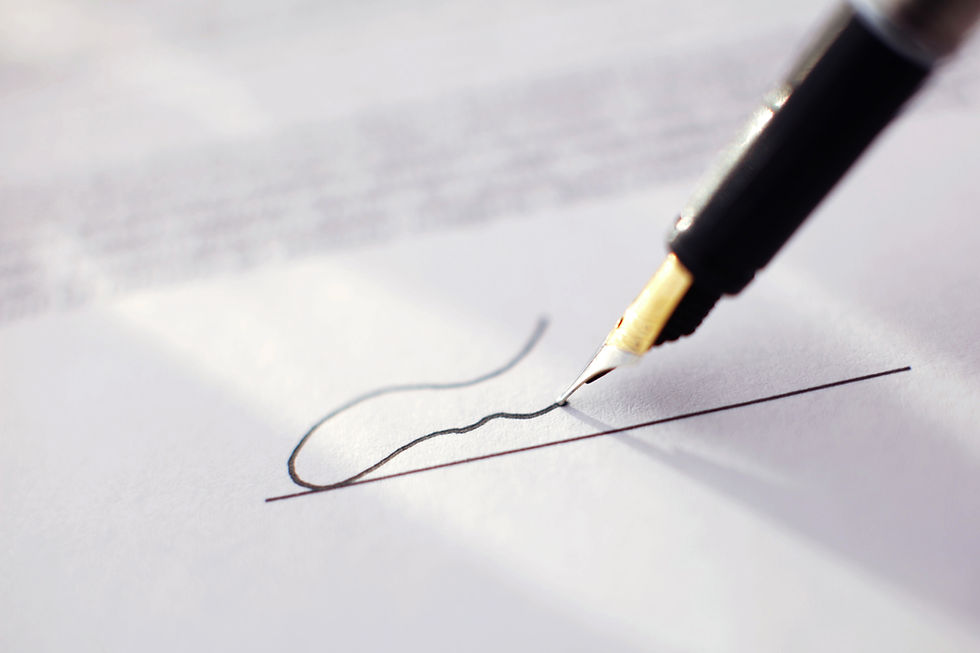
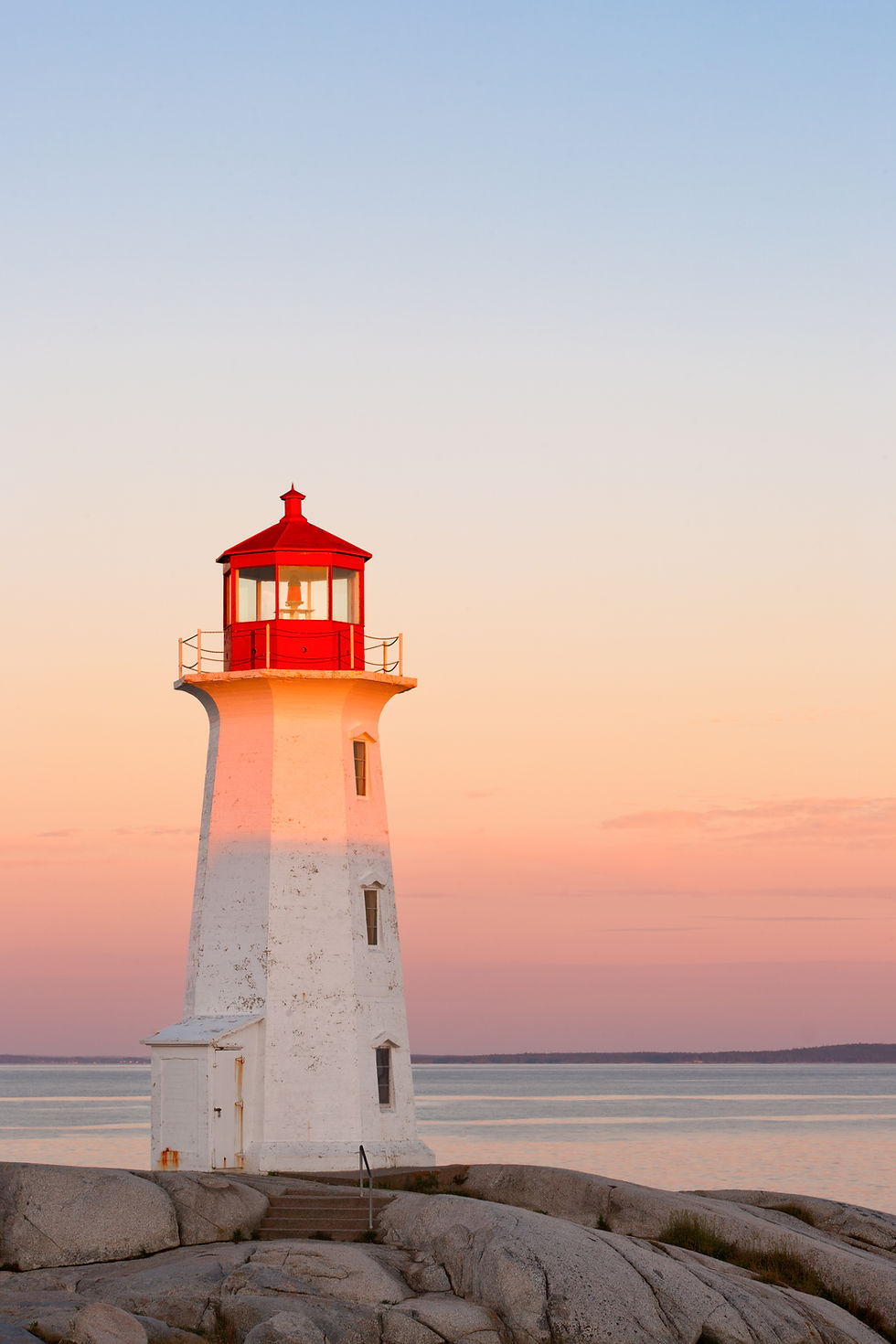
Previous Events of IFAC TC6.3
CPES2022 (Online)
CPES2021 in Moscow (Postponed)
WC2020 in Berlin (Online)
CSGRES2019 in Cheju, South Korea
CPES2018 in Tokyo, Japan
Dead Line: Oct. 31, 2022
Open Invited Track_#71
Advanced Evolutionary Computation for Operation and Planning in Distribution Networks
Proposed by
Hiroyuki Mori
Dept. of Network Design, Meiji University
Nakano-City, Tokyo 164-8525, Japan
E-mail: hmori@meiji.ac.jp
Hirotaka Takano
Dept. of Electrical, Electronic and Computer Eng., Gifu University
Gifu 501-1193, Japan
E-mail: takano@gifu-u.ac.jp
Submission Code: h29pm
IFAC technical committee for evaluation: TC 6.3(Power & Energy)
Abstract
This open invited track provides new methods for distribution network operation and planning with Advanced Evolutionary Computation. In recent years, distribution networks are faced with a lot of uncertainties due to the emergence of Renewable Energy, EV Charging/Discharging, Power Markets, and DR under Smart Grid circumstances. As a result, distribution network operation and planning become much more complicated so that the mathematical formulations result in nonlinear optimization problems with uncertainties. Evolutionary Computation repeatedly makes use of simple rules or heuristics to evaluate highly approximate solutions to a global minimum in nonlinear optimization problems. However, the conventional Evolutionary Computation algorithms such as PSO (Particle Swarm Optimization) and its variants do not necessarily provide good solutions due to the influence of the initial solutions on the final ones in power systems. There is still room for improvement in solution accuracy. Thus, it is necessary to develop the Advanced Evolutionary Computation algorithms which are better than PSO and its variants. Also, well-thought-out approaches are required to deal with the uncertainties.
Detailed description
This track provides new approaches that apply Advanced Evolutionary Computation to distribution network operation and planning. In recent years, renewable energy(RE)has been introduced into distribution networks to reduce carbon dioxide, but the emergence has brought about uncertainties to distribution networks because the generation output is affected by weather conditions. Additionally, there exist other uncertainties such as electricity prices in power markets, load variations, demand response (DR), electric vehicle (EV) charging/discharging, etc. As a result, distribution network operation and planning become much more complicated. To overcome the challenges, new methods are required to deal with distribution networks with uncertainties. This track focuses on Advanced Evolutionary Computation algorithms that are effective for solving nonlinear optimization problems in distribution network operation and planning. Evolutionary Computation is defined as one of the optimization methods which repeatedly makes use of simple rules or heuristics to evaluate highly approximate solutions to a global minimum. However, the conventional Evolutionary Computation algorithms such as PSO (Particle Swarm Optimization) and its variants do not necessarily provide good solutions due to the influence of the initial solutions on the final ones in power systems. There is still room for improvement in solution accuracy. Thus, it is necessary to develop the Advanced Evolutionary Computation algorithms which provide better solutions than PSO and its variants, where by Advanced Evolutionary Computation, we mean high-performance Evolutionary Computation algorithms which are robust for a set of initial solutions in a sense that the obtained solutions are better while the dispersion of the solutions is small for a set of initial solutions. Also, well-thought-out approaches are required to deal with uncertainties. Advanced Evolutionary Computation efficiently allows decision makers to evaluate optimal solutions in distribution network operation and planning. It is challenging to develop new Advanced Evolutionary Computation techniques in distribution network operation and planning. Thus, this track is calling for papers with the following topics:
• Distribution automation with Advanced Evolutionary Optimization
• Loss Minimization with Advanced Evolutionary Optimization
• Network reconfigurations with Advanced Evolutionary Optimization
• State estimation with Advanced Evolutionary Optimization
• Voltage and reactive power control with Advanced Evolutionary Optimization
• Distribution network expansion planning with Advanced Evolutionary Optimization
• Active distribution networks with Advanced Evolutionary Optimization
• Optimal PMU allocation with Advanced Evolutionary Optimization
• Applications of D-FACTS to distribution network operation with Advanced Evolutionary Optimization
• Optimal scheduling of EV charging/discharging with Advanced Evolutionary Optimization
• Probabilistic or Stochastic methods for Distribution automation with Advanced Evolutionary Optimization
• Risk minimization techniques with Advanced Evolutionary Optimization
• Robust optimization for distribution automation with Advanced Evolutionary Optimization
• Parallel or distributed scheme of Advanced Evolutionary Optimization for distribution network operation and planning
• Multi-objective Advanced Evolutionary Computation for distribution network operation and planning
• Optimization of Local Markets with Advanced Evolutionary Optimization, etc.
Open Invited Track_#72
Applications of Advanced Deep Neural Networks to Forecasting Problems in Smart Grid
Proposed by
Hiroyuki Mori
Dept. of Network Design, Meiji University
Nakano-City, Tokyo 164-8525, Japan
E-mail: hmori@meiji.ac.jp
Shoichi Urano
Dept. of Network Design, Meiji University
Nakano-City, Tokyo 164-8525, Japan
E-mail: urano@meiji.ac.jp
Submission Code: f3u93
IFAC technical committee for evaluation: TC 6.3(Power & Energy)
Abstract
This Open Invited Track presents new Deep Neural Networks (DNNs) for forecasting problems in Smart Grid. It is aimed at understanding different DNN models from a standpoint of theoretical background and the applications in Smart Grid. It has important components like Renewable Energy, Power Markets, EVs, DR (Demand Response), Energy Storage Systems (ESSs), Virtual Power Plants (VPPs), etc. As a result, Smart Grid operation and planning become much more complicated and highly nonlinear. Turning our attention to Artificial Intelligence (AI), it is now the third AI boom since the development of Deep Learning in 2006. The use of DNNs has been rapidly spread in the fields of engineering fields due to better performance than the conventional Artificial Neural Networks (ANNs) in terms of model accuracy. On the surface, DNNs might look similar to ANNs, but in practice, DNNs are often different from conventional ANNs. DNNs have been originally developed in the field of image processing, which means that it is not necessarily straightforward to apply DNNs to smart grid operation and planning. Namely, we need to think out new applications by having enough knowledge about DNNs in different case studies. In this invited open track, we focus on forecasting problems in Smart Grid.
Detailed description
This Open Invited Track focuses on applications of DNNs to forecasting variables in Smart Grid that allow decision makers to solve highly nonlinear complicated systems. As DNN models, the following models are widely spread in engineering fields: CNNs (Convolutional Neural Networks), Pre-learning methods with Restricted Boltzmann Machine and Autoencoders, LSTM (Long Short Term Memory) and its variants such as GRU (Gated Recurrent Unit), Residual Networks, GAN (General Adversarial Network), etc. However, DNNs still have potential problems as follows:
-
How to tune up parameters efficiently
-
In conjunction with item1), how to make DNNs more robust for unknown data
-
How to improve model accuracy, etc.
-
How to speed up learning time
It is challenging to handle DNN models with some insights in Smart Grid really well. Therefore, this track is calling for papers with the following topics:
• PV generation output forecasting with Advanced Deep Neural Networks
• Wind power generation output forecasting with Advanced Deep Neural Networks
• Electricity Price forecasting in Power Markets with Advanced Deep Neural Networks
• Load Forecasting Price forecasting with Advanced Deep Neural Networks
• Applications of DNNS to Control of EV Charging/Discharging
• Applications of DNNs to DR (Demand Response)
• Applications of DNNs to Control of Energy Storage Systems (ESSs)
• DNN-based Model Predictive Control for Smart Grid, etc.
Inquiries
Prof. H. Mori, Meiji University, Tokyo, Japan
Secretary of TC6.3